What you will learn
- Master bias detection: Learn to pinpoint potential bias sources in healthcare data and AI algorithms to ensure fair and inclusive healthcare AI applications.
- Build a bias testing framework: Develop a robust framework to evaluate and mitigate bias in AI systems throughout their entire lifecycle, from design to deployment.
- Implement practical strategies: Gain actionable tactics for conducting bias audits, fostering inclusive design, and ensuring ongoing monitoring to maintain fairness in deployed AI solutions.
Session Details
- Intermediate
- 45 minutes
- Includes 15 mins Q&A
- Ethical implications of AI
Session Speaker
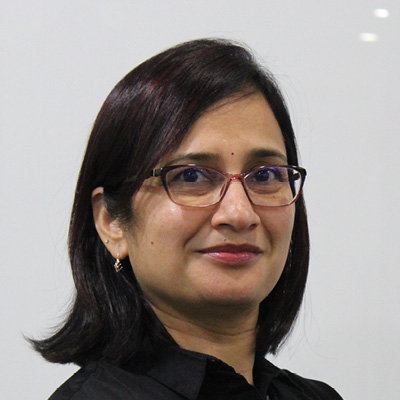
Usha Kandala
Firebucks, India
Usha Kandala is a senior Project Management and Quality Assurance professional with over 20 years of experience in developing and managing software products and product class applications.
She initiated and developed QA practices and release processes in start-up environments, provided leadership and guidance to different teams running multiple product releases, and piloted globally distributed QA teams.
She holds a Master of Liberal Arts degree in Management & Operations from Harvard University, USA; and a Masters degree in Computer Science from Osmania University, India.
She is also an alumnus of Goldman Sachs 10,000 Women Entrepreneurship Program from IIM Bangalore, India.
Stay in the Loop
We want to ensure you never miss important announcements, updates and special offers from EuroSTAR.