What you will learn
- Acquire practical testing approaches- such as adversarial testing, counterfactual evaluations, and detecting training data contamination- to assess the quality of your AI applications, including bias, drift, and robustness.
- Understand the key differences between human reasoning and AI's pattern recognition, and how these differences impact the quality and fairness of your AI applications.
- Develop strategies to refine and adapt your testing practices, ensuring they remain effective as your AI technologies evolve toward more sophisticated reasoning capabilities.
Session Details
- Intermediate
- 45 minutes
- Includes 15 mins Q&A
- Testing the reliability, fairness, and safety of AI models
Session Speaker
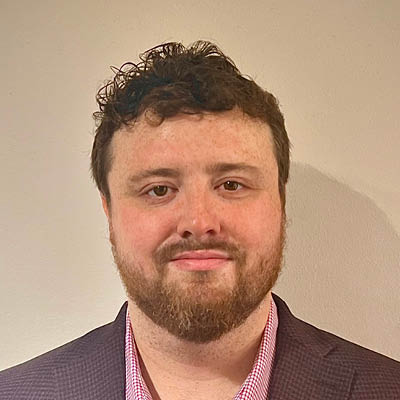
Benjamin Johnson Ward
Curiosity Software, US
Ben has over a decade of experience pioneering innovative testing techniques and tools in the software industry. With a background in behavioural economics from LSE, he explores how unconscious biases influence behaviour and critical thinking in testing.
Throughout his career, Ben has applied this introspective mindset across various quality roles—including developer, product manager, and tester—working with both start-ups and multinational corporations.
He’s helped shape testing techniques at global organisations and is passionate about model-based testing, test data, and recently, AI- especially how we can understand AI systems themselves, and explore new frontiers in AI-driven testing.
Stay in the Loop
We want to ensure you never miss important announcements, updates and special offers from EuroSTAR.